Five theses are intended to show how digitization with artificial intelligence, machine learning, and optimized decision-making is taking private customer business to a new level and how retail banks can use these new opportunities.
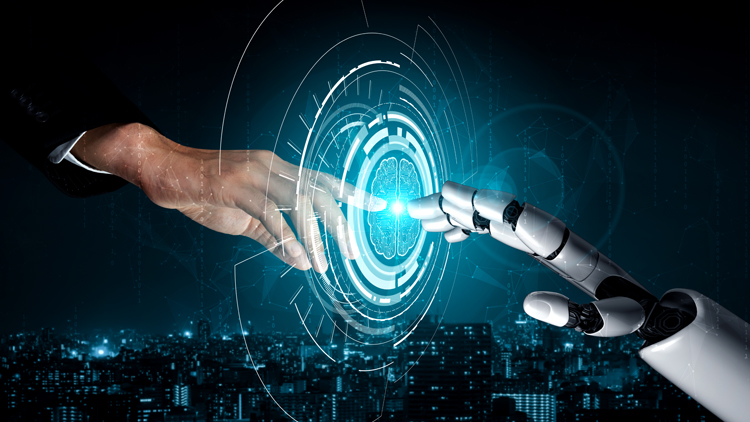
In May 2018, Google CEO Sundar Pichai presented a new technology called Duplex at the Google IO developer conference. This enables Google’s digital assistant to call service providers or restaurants and arrange an appointment with the hairdresser or reserve a table in natural language.
The conversations presented in the demo are indeed impressive, and the filler words inserted by the AI into pauses in speaking make the conversations sound very natural. Irrespective of how market-ready such technologies may be considered: Progress in the development of automatic dialog systems is rapid, and it is foreseeable that such systems are or will be more and more capable of interacting with banks, even in significant parts to take over customers.
This applies in particular to a large number of standardized business transactions that do not require intensive consultation and do not serve to resolve conflicts.
Upheavals in the process automation of banks
Banks are also facing similar upheavals when it comes to process automation: In the first wave of automation 20 to 30 years ago, we saw how certain processes were removed from the branches and transferred to new central units. In “credit factories” such processes were serialized and trimmed for productivity.
With digitization and artificial intelligence, many processes can now be fully automated for the first time – without media breaks and manual intermediate steps. This promises significant cost advantages as well as faster processing and thus a better customer experience. Compared to such fully digital processes, credit factories, as we have known them up to now, seem more like manufactories.
This development is also supported by the fact that the decisions that are necessarily automated in digital processes are constantly improving and regularly surpass the performance of manual decisions and decision strategies developed with classic methods: Methods from operations research such as mathematical optimization enable a quantitative understanding of the tradeoffs between competing companies Goals such as profitability, market share, and risk appetite and thus allow a targeted development of tailor-made decision-making strategies with desired properties. Such strategies are often clearly superior to classic decision rules.
Five Theses on Digitization and AI in Retail Banking
For banks to be successful with digitization and the use of artificial intelligence, some requirements must be met, which I would like to outline below using five theses:
- Digitization requires new skills,
- Digitization requires agile methods,
- AI and operations research open up effectiveness reserves,
- AI requires effort
- AI must be explainable.
Thesis 1: Digitization requires new skills
New technologies always bring new demands on employees. In addition to technical know-how, above all a new mindset is required for the successful automation of processes. The management of digital processes and automated decisions requires employees who view processes – similar to engineering – like a production line. Who thinks about error tolerances, measuring techniques, and more – for example when to intervene in the process and how processes can be controlled?
While you receive feedback from human employees about the success or failure of measures, you cannot expect this kind of “thinking” from the AI at first. Chatbots that don’t have the right answers to customer questions lead to frustrating customer experiences – automatically and over and over again. Employees in process control must therefore keep an eye on all process steps, identify blind spots, and make improvements if necessary.
Digitization will also have an impact on the demands placed on employees in the operational area. When the routine work is taken over by machines, complex and consultation-intensive cases, exceptions, and conflicts are concentrated in Operations – cases that may have previously been escalated to the team leader.
Handling such cases requires more experience and higher qualifications. We are already observing among some market participants that natural fluctuation is being used to reduce the workforce in operational units that are relieved by digital processes. However, when employees are hired, they are significantly more qualified and earn significantly higher salaries than the average employee before the transition.
Thesis 2: Digitization requires agile methods
If communication with customers takes place via digital channels, there is no direct insight into where the crunch is. While employees intuitively adapt their communication to be effective, machines stubbornly follow instructions without providing feedback on what is not working. Banks that are successful with digital processes monitor them closely and adapt them continuously. The results of the individual dialogue and process steps are systematically evaluated and alternative approaches are tested against each other in the champion/challenger procedure.
For this constant learning process, such banks have a cross-functional strategy team that checks the results of the individual process steps, and develops and tries out ideas for improvements in exchange with the specialist departments. A prerequisite for this agile and quantitatively oriented approach is the ability to implement adjustments quickly and largely without the involvement of IT resources.
Therefore, the decision-making technology used must enable employees without programming knowledge to configure rules and communication content independently. The IT department is then used at most for version management, the actual process and rule configuration is the responsibility of the departments.
Thesis 3: AI and operations research open up effectiveness reserves
Which credit offers or limits a customer receives, which marketing initiatives are promising for a target group, or how intensively the receivables management approaches customers – the corresponding decision-making strategies are often developed based on experience. Although mathematical models are often used to segment the portfolio, mathematical methods are often used insufficiently when allocating decisions, measures, or prices to these segments.
This leads to significant opportunity costs. I expect that methods from operations research will increasingly be used in the development of pricing strategies, but also in operational processes, as has been the case in other industries for decades. With mathematical decision optimization, for example, the dependencies of sales, profitability, and risk targets can be quantitatively understood so that decision strategies can be optimally tailored to competing targets.
Not using these methods is expensive: I estimate that banks lose a three-digit million amount every year with installment loans alone because not enough mathematical methods are used when developing pricing strategies.
Read Also: Explore the Exciting World of AI Virtual Girlfriends: Your Fun Companion
Thesis 4: AI requires effort
There is an aura of effortlessness about machine learning and artificial intelligence: problems solve themselves. This assessment is incorrect. Many underestimate that the introduction of AI requires good preparation and regular monitoring and readjustment. This begins with the selection of the method: not every algorithm is suitable for every problem. And a model is only as good as the underlying data.
Accordingly, careful data preparation and cleansing is essential. In order to achieve real added value, a deeper understanding of one’s own goals as well as the methods and possibilities is absolutely necessary. Where is the value in a business process and what decision has the most impact? What knowledge and predictions could help improve this decision? The answers to these questions will also have to be provided by human employees in the future.
Thesis 5: AI must be explainable
When using AI, automated decisions are made en masse. This harbors the risk that errors and unwanted bias will be replicated automatically. A decision logic that represents a black box therefore harbors incalculable risks. It is important to understand how the models used work, and the data characteristics that dominate a decision must be explainable and plausible – both at the global level and for the individual decision.
However, according to market research firm Corinium’s State of Responsible AI study commissioned by FICO, 65 percent of the companies surveyed cannot explain how AI decisions or predictions are made. Only a fifth actively monitor models for fairness and ethics. These are worrying numbers.
However, the explainability of AI-based decisions does not only result from the principles of good corporate governance: In discussion papers from BaFin and the EBA, regulation is already emerging that requires explainability at least for risk-relevant decisions. We probably won’t have to wait much longer for such regulations in analogy to the MARisk (“minimum requirements for artificial intelligence”). The need to use explainable methods therefore arises for reasons of investment security.
Conclusion: AI can help to develop potential in retail banking
Artificial intelligence and digitization promise to significantly improve the traditionally difficult cost structure in the low-margin private customer business. Machine learning, language processing, robotics – the artificial intelligence kit offers a range of smart tools that machines can use to act autonomously.
In order not to fall behind in the face of rapid technological developments, financial institutions need to know where their digitization journey is headed and how they want to use the innovative technologies in a focused manner.
As with all automation projects, it is therefore important to use these tools judiciously. Because even intelligent technologies cannot repair mediocre processes as if by magic. It is therefore worth focusing on the decisions and processes that deliver the greatest value for your own organization. But then artificial intelligence is not just another buzzword, but can open up completely new potential in retail banking.